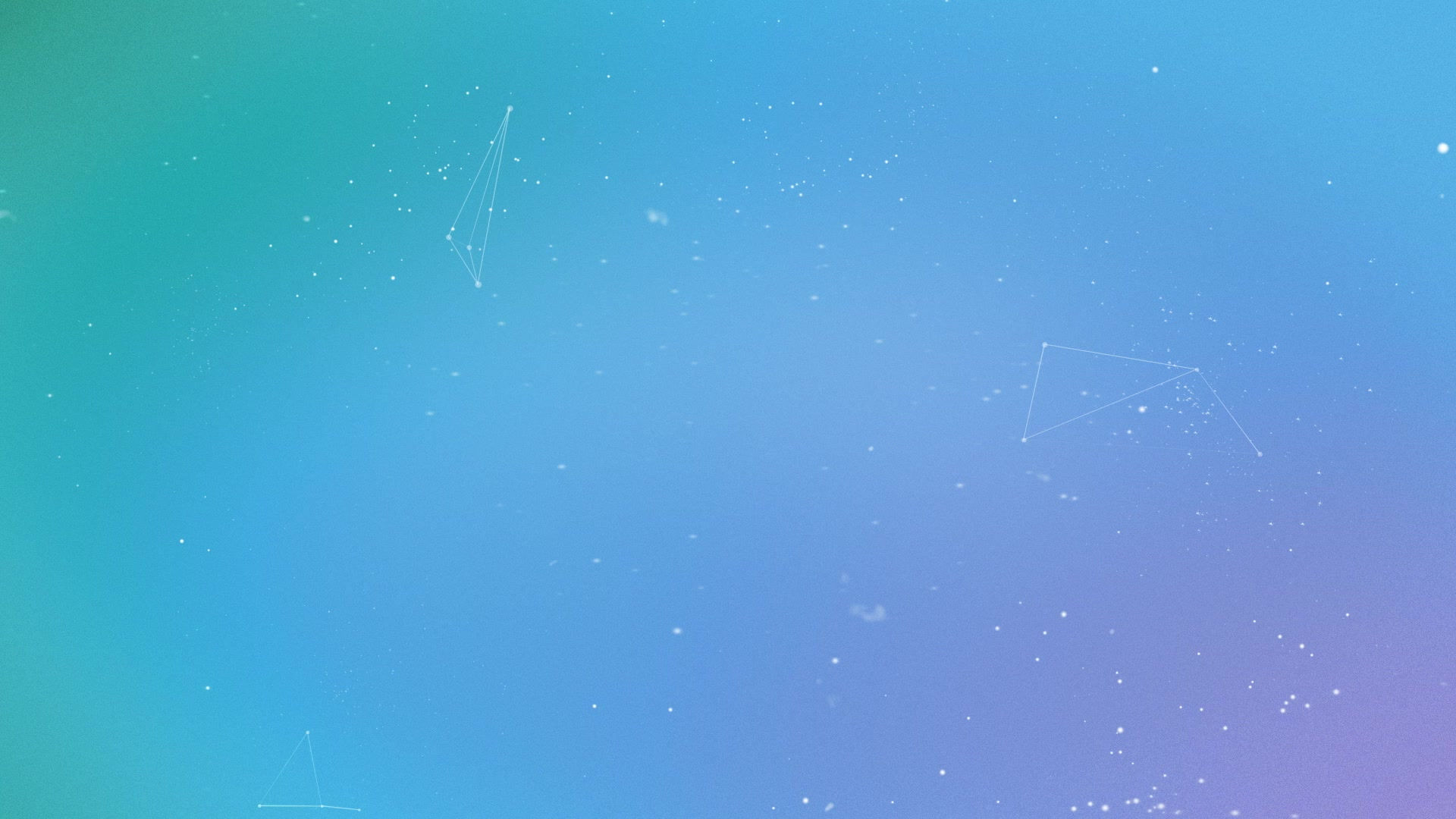

STATSCALE SEMINARS
PREVIOUS SEMINAR - 10th February 2023
Zifeng Zhao (University of Notre Dame)
​
​
Title: High-Dimensional Dynamic Pricing under Non-Stationarity: Learning and Earning with Change-Point Detection
Abstract - We consider a high-dimensional dynamic pricing problem under non-stationarity, where a firm sells products to $T$ sequentially arrived consumers that behave according to an unknown demand model that may change at unknown locations over time. The demand model is assumed to be a sparse high-dimensional generalized linear model~(GLM), allowing for a feature vector in $\mathbb R^d$ that encodes products and consumer information. To achieve optimal revenue~(i.e.\ least regret), the seller needs to learn and exploit the unknown GLM model while at the same time monitoring for potential change-points~(CP). To tackle such a problem, we first design a novel penalized likelihood based online CP detection algorithm for high-dimensional GLM, which is the first in the CP literature that achieves optimal minimax localization error rate for high-dimensional GLM. A CP detection augmented dynamic pricing policy named CPDP is further proposed, which achieves a regret of order $O(s\log(Td)\sqrt{U_T T})$, where $s$ is the sparsity level and $\Upsilon_T$ is the number of CP. Somewhat surprisingly, this regret order is independent of the magnitude of the change size. A matching lower bound is further provided to show the optimality of CPDP (up to logarithmic factors). In particular, the optimality w.r.t.\ the number of CP $\Upsilon_T$ seems to be the first in the dynamic pricing literature, and is achieved via a novel accelerated exploration mechanism. Extensive simulation experiments and a real data application on online lending illustrate the efficiency and practical value of the proposed policy.
​
​
RETURN TO SEMINAR HOMEPAGE